The Cramer group at Rensselaer Polytechnic Institute has a longstanding history of contributions to the field of column modeling over the course of over three decades. One of the earliest contributions was the development of the steric mass action (SMA) model [1], describing adsorption of ion exchange chromatography with applications to displacement.
Beyond this, efforts over the past decades have led to the development and application of models for ion exchange chromatography (IEC) [1,2], immobilized metal affinity chromatography (IMAC) [3], hydrophobic charge-induction chromatography (HCIC) [4,5], hydrophobic interaction chromatography (HIC) [6,7], and in more recent years—multimodal chromatography [8–12]. These endeavors have been centered around both the fundamental and the applied aspects of chromatographic science—using first-principles modeling approaches. Some key applications of modeling have included process optimization and control , connection with protein surface properties , and high-throughput chromatographic modalities [11–14]. Results have been published to a number of reputable scientific journals including, but not limited to, Journal of Chromatography A, Biotechnology Progress, Biotechnology and Bioengineering, AIChE Journal, and Chemical Engineering Science, and Separation Science and Technology. Column modeling research projects in the group have been funded by an array of sources and performed under the supervision/collaboration of diverse groups—including government agencies (NSF, NIH, and NIIMBL), biopharmaceutical companies (Merck, MedImmune/AstraZeneca, and Genentech), and academic institutions (North Carolina State University and University of Delaware).
Selected figures from recent publications
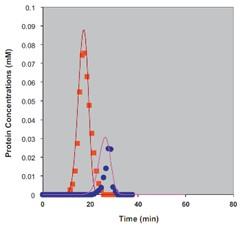
Nagrath et al. – 2011 Journal of Chromatography A – Characterization and modeling of nonlinear hydrophobic interaction chromatographic systems. Shown below: prediction of ammonium sulfate gradient elution behavior in Phenyl 650M HIC system for lysozyme and lectin model proteins [7].
Modeling of HIC ammonium sulfate gradient elution. Squares: lysozyme; Circles: lectin.
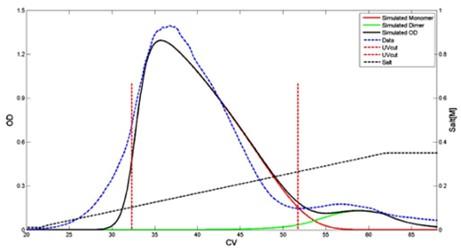
Sejergaard et al. – 2014 Biotechnology Progress – Model-based process development for the purification of modified human growth hormone using multimodal chromatography. Shown below: prediction of modified human growth hormone elution behavior using Capto Adhere resin with arginine hydrochloride eluent [9].
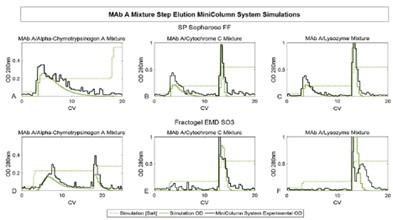
Keller et al. – 2017 Journal of Chromatography A – Understanding operational system differences for transfer or miniaturized chromatography column data using simulations. Shown below: predictions of MiniColumn step elution experiments for two cation exchange resins and various mixtures of monoclonal antibodies and model proteins [14].
Prediction of MiniColumn step elution experiments. Green: simulated elution profiles; Black: experimental elution profiles.
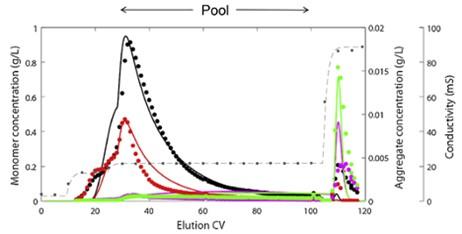
Zhang et al. – 2019 Journal of Chromatography A – Mechanistic modeling based process development for monoclonal antibody monomer-aggregate separations in multimodal cation exchange chromatography. Shown below: predictions of step gradient elution profiles of monoclonal antibody monomer and aggregate species [10].
Prediction of step elution profiles for monoclonal antibody monomer and aggregate species on a multimodal-cation exchange resin. Black: monomer; Red: HMW1; Magenta: HMW2; Green: HMW3.
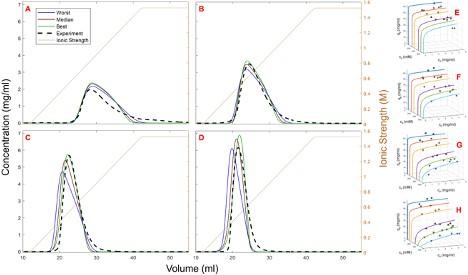
Altern et al. – 2022 Journal of Chromatography A – Isotherm model discrimination for multimodal chromatography using mechanistic models derived from high-throughput batch isotherm data: (I) Multimodal cation-exchange (Submitted for review). Shown below: prediction of complex (pH-dependent) linear gradient elution behavior model in multimodal cation-exchange chromatography using an extended SMA with isotherm parameters obtained from fitting batch (microtiter plate) adsorption experiments across a range of pH, salt, and protein concentrations [11].
Prediction of multimodal cation-exchange elution behavior under various pH conditions. A) pH 5.3; B) pH 5.6; C) pH 5.9; D) pH 6.2. Solid blue lines: lowest scoring prediction; Solid brown lines: median scoring prediction; Solid green lines: best scoring prediction; Dotted black lines: experiment. E) pH 5.0; F) pH 5.25; G) pH 5.5; H) pH 6.0.
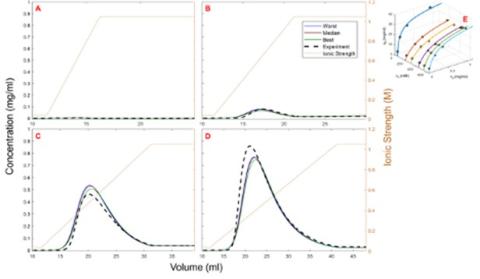
Altern et al. – 2022 Journal of Chromatography A – Isotherm model discrimination for multimodal chromatography using mechanistic models derived from high-throughput batch isotherm data: (II) Multimodal anion-exchange (Submitted for review). Prediction of complex (gradient slope-dependent) linear gradient elution behavior in multimodal anion-exchange chromatography using an extended SMA model with isotherm parameters obtained from fitting batch (microtiter plate) adsorption experiments across a range of salt and protein concentrations [12].
Prediction of multimodal anion-exchange elution behavior under various gradient slopes. A) 5 CV gradient length; B) 10 CV gradient length; C) 20 CV gradient length; D) 30 CV gradient length. Solid blue lines: lowest score predictions; Solid brown lines: median score predictions; Solid green lines: best score predictions; Dotted black lines: experiment. E) batch isotherm fit at pH 8.0.
Selected Reference :
[1] Brooks CA, Cramer SM. Steric mass-action ion exchange: Displacement profiles and induced salt gradients. AIChE J 1992;38:1969–78.
[2] Gallant SR, Vunnum S, Cramer SM. Modeling Gradient Elution of Proteins in Ion-Exchange Chromatography. AIChE J 1996;42:2511–20. https://doi.org/10.1002/aic.690420911.
[3] Vunnum S, Gallant SR, Kim YJ, Cramer SM. Immobilized metal affinity chromatography: Modeling of nonlinear multicomponent equilibrium. Chem Eng Sci 1995;50:1785–803. https://doi.org/10.1016/0009-2509(95)00038-7.
[4] Ghose S, Hubbard B, Cramer SM. Protein interactions in hydrophobic charge induction chromatography (HCIC). Biotechnol Prog 2005;21:498–508. https://doi.org/10.1021/bp049712+.
[5] Ghose S, Hubbard B, Cramer SM. Evaluation and comparison of alternatives to Protein A chromatography. Mimetic and hydrophobic charge induction chromatographic stationary phases. J Chromatogr A 2006;1122:144–52. https://doi.org/10.1016/j.chroma.2006.04.083.
[6] Xia F, Nagrath D, Cramer SM. Modeling of adsorption in hydrophobic interaction chromatography systems using a preferential interaction quadratic isotherm. J. Chromatogr. A, vol. 989, Elsevier; 2003, p. 47–54. https://doi.org/10.1016/S0021-9673(02)02047-2.
[7] Nagrath D, Xia F, Cramer SM. Characterization and modeling of nonlinear hydrophobic interaction chromatographic systems. J Chromatogr A 2011;1218:1219–26. https://doi.org/10.1016/j.chroma.2010.12.111.
[8] Karkov HS, Sejergaard L, Cramer SM. Methods development in multimodal chromatography with mobile phase modifiers using the steric mass action model. J Chromatogr A 2013;1318:149–55. https://doi.org/10.1016/j.chroma.2013.10.004.
[9] Sejergaard L, Karkov HS, Krarup JK, Hagel ABB, Cramer SM. Model-based process development for the purification of a modified human growth hormone using multimodal chromatography. Biotechnol Prog 2014;30:1057–64. https://doi.org/10.1002/btpr.1923.
[10] Zhang L, Parasnavis S, Li Z, Chen J, Cramer S. Mechanistic modeling based process development for monoclonal antibody monomer-aggregate separations in multimodal cation exchange chromatography. J Chromatogr A 2019;1602:317–25. https://doi.org/10.1016/j.chroma.2019.05.056.
[11] Altern SH, Lyall J, Kocot AJ, Welsh JP, Burgess S, Kumar V, et al. Isotherm model discrimination for multimodal chromatography using mechanistic models derived from high-throughput batch isotherm data: (I) Multimodal cation-exchange (Submitted for review). J Chromatogr A 2022.
[12] Altern SH, Lyall JY, Kocot AJ, Welsh JP, Burgess S, Kumar V, et al. Isotherm model discrimination for multimodal chromatography using mechanistic models derived from high-throughput batch isotherm data: (II) Multimodal anion-exchange (Submitted for review). J Chromatogr A 2022.
[13] Keller WR, Evans ST, Ferreira G, Robbins D, Cramer SM. Use of MiniColumns for linear isotherm parameter estimation and prediction of benchtop column performance. J Chromatogr A 2015;1418:94–102. https://doi.org/10.1016/j.chroma.2015.09.038.
[14] Keller WR, Evans ST, Ferreira G, Robbins D, Cramer SM. Understanding operational system differences for transfer of miniaturized chromatography column data using simulations. J Chromatogr A 2017;1515:154–63. https://doi.org/10.1016/j.chroma.2017.07.091.