Machine learning (ML) using big data is a powerful tool for predicting protein surface properties like hydrophobicity and binding affinity. Large amounts of data generated from diverse experimental techniques and molecular simulations provide an opportunity to build ML-based models capable of accurately predicting these properties, as well as identifying interaction hot spots and binding pockets. Proteins have distinct chemical and topographical features, which need to be encoded suitably in order to utilize the benefits of machine learning algorithms; hence, geometric data representations like point clouds, graphs, and voxel-based representations have demonstrated effectiveness in interaction prediction tasks. Moreover, generative models, such as GANs and VAEs, offer a platform to predict protein structure properties by generating new structures and interpolating between known structures with specific properties.
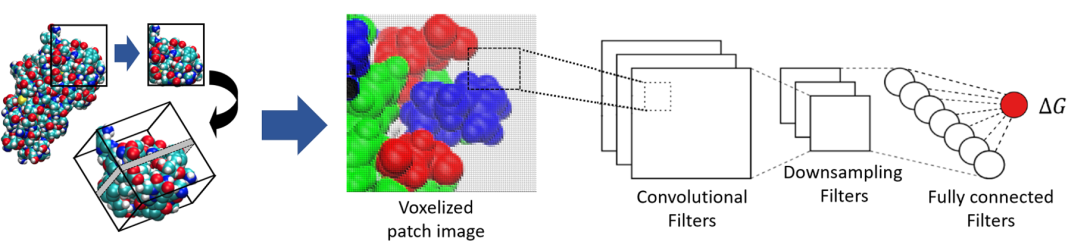
We have utilized a voxel based convolutional variational autoencoder (VAE) as a generative model to map tertiary patch inputs onto a continuous latent space. These features depict patch topography and chemistry in a low-dimensional format and enable generation of new protein patches and perturbation of existing patches. This approach allows us to examine functional 3D surface patches in a latent space characterized by chemistry and topography, which enables optimization routines. Concept vectors defining essential attributes of data withinthe latent space have been studied before, and we propose that similar concept vectors for protein tertiary patches may describe important structural or chemical features.
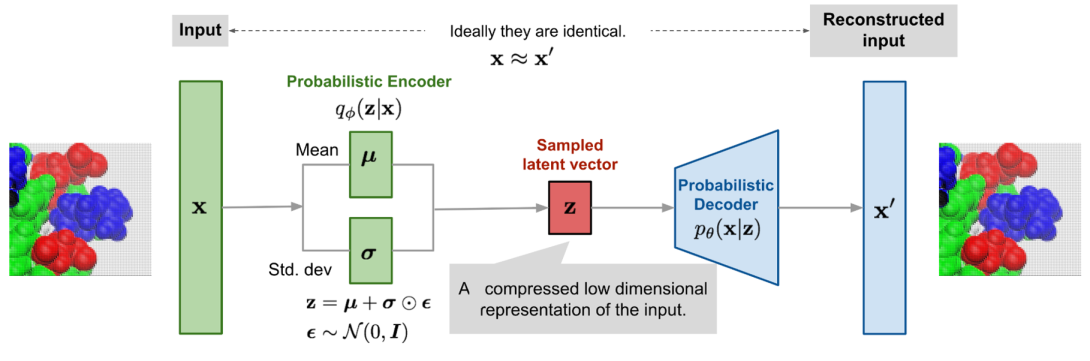
We have demonstrated that the "latent space walk“ between two patch vectors produced highly realistic patches that maintain continuity in pattern and chemistry. The latent space walk represents a smooth transition in the structure and chemistry of patches located at different points on the variational latent space. Our findings indicated that these latent vector embeddings were correlated with patch density and relative solvent exposure.M
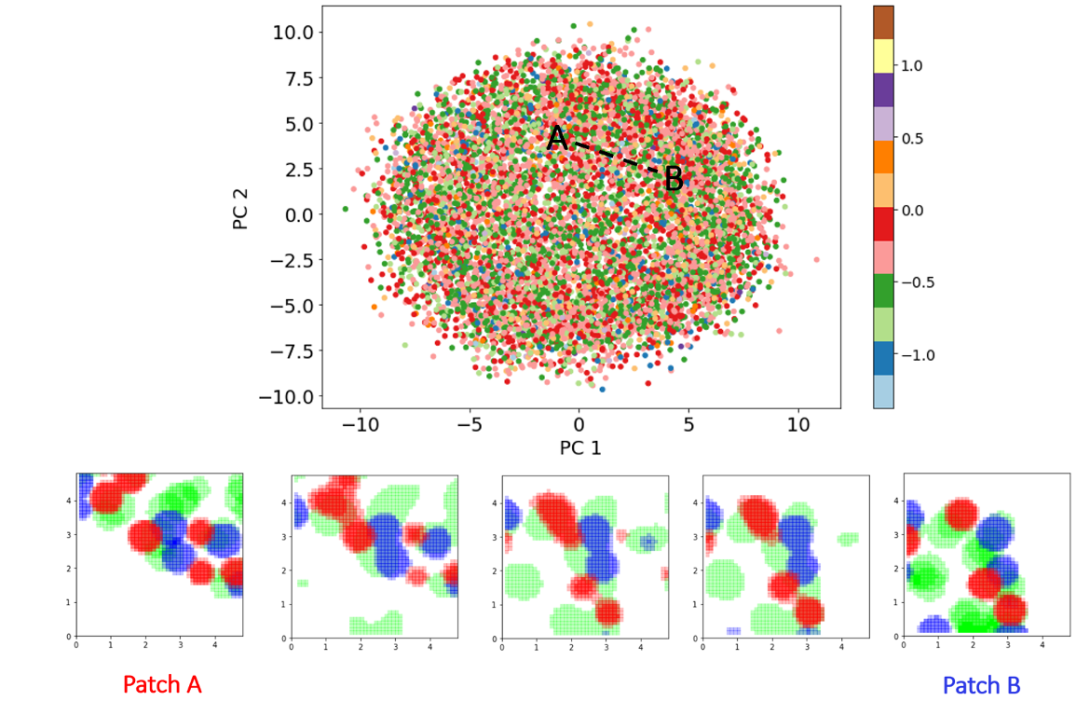
The variational autoencoder when trained jointly with a property prediction regression model can be used to predict a specific property of interest. When trained jointly, the latent space rearranged based on the gradient of the property being predicted. This is achieved by introducing a regressor network that predicts the label of each input patch, and a regularization term that encourages the latent representation to be predictive of the label. By jointly optimizing the reconstruction loss, the regression loss, and the regularization term, the VAE can learn a more informative latent representation of the data that can be used to predict the surface properties of unlabeled protein patches.
(Cr: Dr. Imee Sinha)

Selected References:
1.Sinha, Imee. Characterization of Protein Surface Hydrophobicity Using Molecular Dynamics Simulations and Deep Learning. Diss. Rensselaer Polytechnic Institute, 2022.
2.Kingma, Diederik P., and Max Welling. "Auto-encoding variational bayes." arXiv preprint arXiv:1312.6114 (2013).
3.https://lilianweng.github.io/posts/2018-08-12-vae/